Quantitative risk assessment (QRA) is a practical approach for evaluating and analyzing risk. Some proponents of QRA argue that it is the ultimate approach for measuring risk. It is, however, not without its limitations.
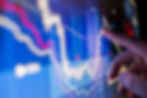
The limitations of QRA
As valuable as it is, there are some fundamental limitations to QRA, including the following:
Quality of data
Availability of data
Applicability of data
The reliance on historical data
Counterparty risk
Human factors
The illusion of precision
Causality and independence
Quality of data
The expression from computer programming 'garbage in, garbage out (GIGO) is particularly relevant for QRA. Any errors, limitations, or biases in the data set used for QRA will have a compounding effect.
Minor imperfections in data sets or algorithms will multiply with each iteration of the data and may lie unnoticed for many years, often until it is too late.
Availability of data
The more available data, the higher the likelihood it will be accurate. The law of large numbers implies that our forecasts or assessments will become more accurate with more information. While this is not strictly true, as a general rule, we can say that the more information we have available (provided it is carefully selected), the more reliable our outputs will be.
There are many issues with this, however. In most situations, there will be some information already available, but it is rare to find all the information needed for quality analysis. That additional information is unlikely to be free.
Determining how much information is needed, how to acquire the missing elements, and how much that will cost in time, money, or resources requires subtle judgments by skilled human operators.

Colin Powell, a retired United States military officer and former U.S. Secretary of State, is known for his 40-70 rule in decision-making. This is about making decisions when we have between 40% and 70% of the information we need to decide the best solution. Waiting to have more than 70% of the data is likely to delay the decision and mean taking action too late unnecessarily.
Acquiring more of the potentially helpful information than we need (70%) is costly in terms of money and missed opportunities. Having inadequate information (notionally less than 40%) virtually guarantees that the outputs will be unreliable.
Applicability of data
One inherent limitation of the law of large numbers is that the outputs are generally only reliable when applied broadly. An actuarial study of life expectancy can provide helpful information about a person's likely lifespan. But that information does not reliably tell how long an individual will live.
Similarly, quantitative risk models only apply to weather events or financial portfolios when looking at large data sets. They provide some guidance about individual assets or locations but cannot definitively forecast or rate individual outcomes.
Focus on historical data
Another core limitation of QRA is that it relies on historic data. Even real-time data is ephemeral. Within moments, it becomes historical information. Whether that is stock market volatility, life expectancy, extreme weather events, or the number of house fires in a particular location, the prime dataset is historical. While this is a sound basis to commence analysis, it provides no guarantee of the future.
Any changes in the underlying assumptions about that data continuing or changing introduce uncertainty into the projected outcomes. Climate change is progressively showing our extreme weather forecasts to be unlike our historical records. Similarly, a change in building materials, electrical standards, or mandatory smoke detectors can quickly change the incidence of house fires in a city.
Quantitative risk analysis can be used with projections such as decision trees or Monte Carlo models. The inherent limitations of these approaches, however, should be obvious. Without sound historical or experimental data, such projections are bound by the data's limitations and assumptions introduced at the design stage.

Even in relatively stable systems such as aviation, the discovery of a design flaw in an aircraft type can result in hundreds of aircraft being grounded overnight, with a resulting impact on thousands of flights and millions of people, as well as the profitability of the aviation sector at large.
Counterparty risk
The story of Long Term Capital Management (LTCM) is a cautionary tale of the limitations of QRA. Founded and operated by geniuses with PhDs and Nobel Prizes, LTCM conducted extraordinarily profitable options trades based on quantitative models.
For several years, they beat the market with annual returns of approximately 40% per annum. And then, one day, in August 1998, Russia devalued the rouble and declared a moratorium on 281 billion roubles ($13.5 billion) of its Treasury debt.
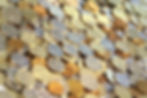
LTCM believed that its derivatives positions in Russian bonds were hedged by selling to the extent that a default on the bonds would undeniably lead to a collapse of the currency. They believed a profit could still be made in the foreign exchange market that would outweigh the losses. Unfortunately, the banks on which the strategy of the rubble hedge relied collapsed too, and the Russian government prevented further trading in its currency.
The financial models of LTCM were near perfect. But they relied on their counterparties' continued viability and goodwill—something that no model can guarantee, however precise it may seem.
Human Factors
Quantitative risk management systems are managed and designed by humans. And humans have emotions, cognitive biases, and individual decision-making perspectives. Typical QRA tools involve a 'fail-safe' or early warning system to prevent a repeat of the LTCM catastrophe or its equivalent.
These early warning signals, however, are available to everyone. Others can also see any stop-loss or early warning system that an organization can detect.
The Electronic Herd is a term coined by Thomas Friedman in his book The Lexus and the Olive Tree. This herd gathers in key global financial centers such as Wall Street, Hong Kong, London, and Frankfurt. The attitudes and actions of the Electronic Herd and the markets can have a considerable impact on nation-states today, even to the point of triggering the downfall of governments. Who ousted Suharto in Indonesia in 1998? It wasn't another state; it was the markets, withdrawing their support for, and confidence in, the Indonesian economy.
Today's global marketplace is an Electronic Herd of often anonymous stock, bond, and currency traders and multinational investors, connected by screens and networks. This electronic herd concept applies equally to any risk management scenario.
The spread of real or fake news can, for example, devastate the economy, infrastructure, or the uptake of and support of vaccinations during a pandemic. Such scenarios can be catered for in quantitative risk analysis but at the cost of resources, complexity, and accuracy.
The illusion of precision
Risk analysis with abundant resources and historical data that provides results to four decimal points may appear robust and conclusive. Indeed, it will be helpful and better than no analysis at all. But it will still only provide estimates of probability and likely consequences.
Even if this involves probability distributions and confidence intervals, such illusions of certainty are still probabilistic with some (often unknown) level of uncertainty and ambiguity.
A 5% chance of rain suggests that it is unlikely to rain but does not preclude the possibility of rain. Declaring a 4.9783% chance of rain does nothing to improve the accuracy of the forecast.
Even with a probability distribution, there is no guarantee that all the potential extreme outliers have been included. Nor that the most likely outcomes will eventuate, and adding more decimal points does nothing to change that.
Causality and independence
Another challenge for QRA is that initiating events in a causal chain are usually assumed to be mutually exclusive. While this assumption simplifies mathematics, it may not match reality. Most accidents in well-designed systems involve two or more low-probability events occurring in the worst possible combination. When people attempt to predict system risk, they often explicitly or implicitly assume independence and come out with impossibly small numbers when, in fact, the events are dependent.
This dependence may be related to common systemic factors that do not appear in an event chain. Machol calls this phenomenon the Titanic coincidence. Many factors that contributed to the loss of life during the sinking of the Titanic may seem independent. Believe in the 'unsinkability' of the Titanic due to incorrect risk analyses likely contributed to the catastrophe.
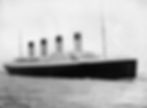
Factors such as the captain going too fast for existing conditions, an inadequate watch for icebergs, insufficient lifeboats, the absence of lifeboat drills, and inadequate arrangements for lifeboats can all be attributed to the assumption of unsinkability. As a result, assuming independence can lead to a significant underestimation of the actual risk.
A related problem in QRA is that the emphasis on failure events-design errors are usually omitted and only come into the calculation indirectly through the probability of the failure event. In the 2008 financial markets collapse, for example, many organizations had dynamic quantitative risk models yet failed spectacularly.
Investors such as Michael Burry were considering the risks inherent in the system design of the bond and real estate derivative markets. They understood the risk inherent in the assumption of ever-rising real estate prices; a risk compounded daily by creating arcane, artificial securities loosely based on piles of doubtful mortgages.
Many methods for quantifying risk are based on combining probabilities of individual component failures (e.g., FMEA). QRAs that rely on an assumption of mutually exclusive events should be applied with great caution, particularly to complex systems controlled by software or by humans making cognitively difficult decisions. Very few techniques can adequately incorporate management and organizational factors, such as culture and human error, into quantitative risk analysis.
How to manage these limitations
Several strategies and risk management tools can mitigate the limitations of quantitative risk analysis. Techniques such as scenario modeling, think tanks, business case development, human factors analysis, decision trees, and root cause analysis can provide valuable insights. Insights that can inform and modify quantitative studies if applied correctly.
The best outputs of quantitative risk assessments are likely to be probability distributions, sensitivity analysis, decision trees, or tornado diagrams. These provide visual probabilistic models that inform decision-making rather than make decisions automatically. Before this, a critical element of any quantitative risk assessment is defining the risks correctly, which can be, surprisingly, often overlooked.
If you're looking for more insights on these, you might find some of these links helpful: